When “Good Enough” Data Isn’t: Why AI Success Demands a Clean Data Diet
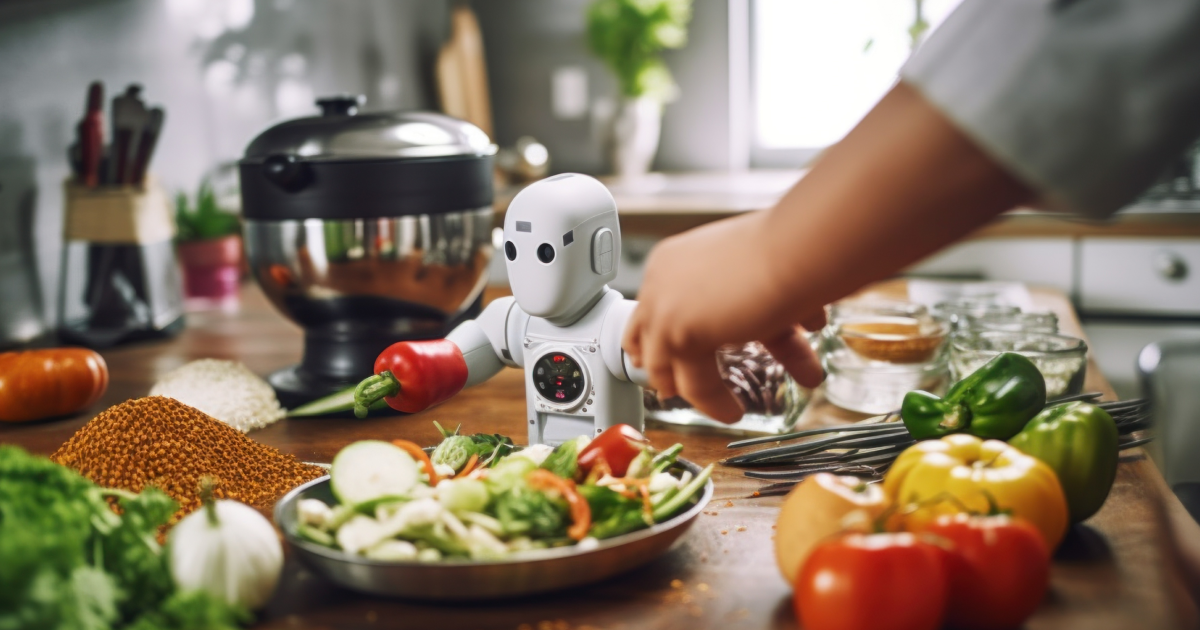
The real estate industry has always been a quick mover when it comes to dealmaking, despite its bearish attitudes towards tech. In a business driven by relationships, reputation, and responsiveness, there’s some tolerance for making big decisions with less-than-perfect information, but that’s rapidly changing.
As artificial intelligence (AI) adoption grows, the impact of incomplete or imperfect data becomes wider-reaching and more dangerous. Humans in decision making roles have enough context and experience to make judgment calls with limited information, but even the smartest AI can deliver bad decisions – or hallucinate– when missing the data it needs.
AI Use Cases for Real Estate
The simplest way to consider AI as a tool without getting overwhelmed is to isolate the tasks that need doing that require reviewing information to make a decision.
Here are some examples of real estate work easily accomplished with AI, along with data types you would need to provide:
- Property valuation
- Construction costs
- Recent property transaction data
- Sales data for comparable properties by asset class, geographic location, etc.
- Risk assessment
- Environmental assessments and survey data
- Title and ownership history
- Indicators of market volatility.
- Lease management
- Existing lease agreements and terms
- Tenant payment history
- Maintenance and repair records
- Relevant market data.
- Tenant screening
- Tenant credit scores or financial statements
- Rental payment history
- Previous landlord references
- Employment history.
- Portfolio management
- Property financial statements
- Age and condition of all properties
- Lease expiration dates and renewal options
- Market data and growth forecasts.
- Operational efficiency
- Maintenance and repair schedules
- IoT sensor data for connected systems and devices
- Utility consumption data
- Vendor and contractor records and performance data
- Invoice and payments history.
- Compliance
- Environmental compliance reports
- Tax assessment and reporting data
- Fair housing and anti-discrimination records.
- Environmental Sustainability
- Energy consumption data
- Utility rates over time
- Sustainability certification records or certifications
- Environmental impact assessments.
This is where most organizations run into trouble. Too much of the data they rely on exists in disjointed pockets of institutional knowledge spread across digital files, cabinets full of paper, or individual employee memory.
Humans learn to compensate for missing info with collaboration and intuition. Your tech will need a leg up to prevent creating inefficiencies, inaccuracies, or introducing new potential risks.
How to Clean Up Your Data for AI Projects
For the purpose of operationalizing by way of AI, the bar for clean, usable data is availability, readability, completeness, reliability, and consistency. Timeliness increases in importance when quick decisions are required.
Here’s a standard approach to getting your data AI-ready:
- Audit:
- Identify all available data sources, their reliability and usefulness
- Identify all business processes around data use and collection
- Identify data gaps that prevent automation
- Identify inconsistencies, inefficiencies, and workarounds due to system or data limitations
- Focus:
- Define the questions you want to be able to answer or decisions you’d like to make automatically and map out paths or flows from data point to decision
- Decide what to solve for and don’t look for a one-size-fits-all solution, focus on one business problem at a time
- Centralize:
- Select a single source of truth like a CRM or ERP or other database where your data can be maintained and updated
- Define a process for importing data from other sources when necessary
- Standardize:
- Prune what’s not necessary, standardize naming and numbering conventions
- Remove duplicate data – this is one of the biggest problems for accuracy and consistency down the road
- Define a schema for your data management – this could be as simple as a spreadsheet to indicate the name, type, and purpose of each field in a database record, and can help solidify decision making and prevent unnecessary complexity as your needs evolve
- Restrict access to key data
- Educate:
- Before you train your AI, train your employees, your vendors, your partners, anyone who interacts with your data
- Everyone should have a shared understanding of how things work and why, how to update information, and whose responsibility it is to keep it up to date
- Schedule regular reviews and re-training sessions with your team to keep everyone on the same page
- Select:
- Now you’re ready to consider the right AI solution for your needs—if it seems to be late in the process, you’re underestimating the size of the gap between what AI can do for your business and what’s required to succeed
- The better defined your problem, parameters, and available data, the easier it will be to choose the right level of intelligence and automation for your project; you might find an AI solution already exists specifically for lease abstraction or invoice processing
- Make the most of available models and resources by balancing what will be optimal for how you intend to use your data and will integrate into your existing tech stack and processes without needless disruption – doing the cleanup work up front will make this easier
- Train & Review:
- Once you start training AI to operationalize your data, you’ll find ample opportunities to refine and perfect what it can do, but this part will remain ongoing
- The best AI, or the best AI for each job, will continue to show improvement with more cycles and as more data becomes available – that’s what separates it from simple workflows and flowcharts or templates
- Make regular review of results and expectations a part of your AI management strategy and enjoy the fruits of improved speed and efficiency across your business operations
As our industry evolves and adopts new technologies, the commitment to clean data becomes increasingly vital. Shortcuts taken in the past to expedite processes can transform into significant impediments when AI adds unprecedented speed and scalability. Every organization goes through these cycles, but those that invest in clean data will reap lasting dividends in terms of efficiency, accuracy, and innovation.
This Week’s Sponsor
PredictAP automates invoice capture for Yardi Payscan using proprietary AI. It eliminates manual data entry and improves AP outcomes without disrupting existing workflows. PredictAP is a Yardi Standard Interface Partner with US and EU API access, delivering seamless integration, and rapid time to value. Learn more at www.predictap.com.
Read Next
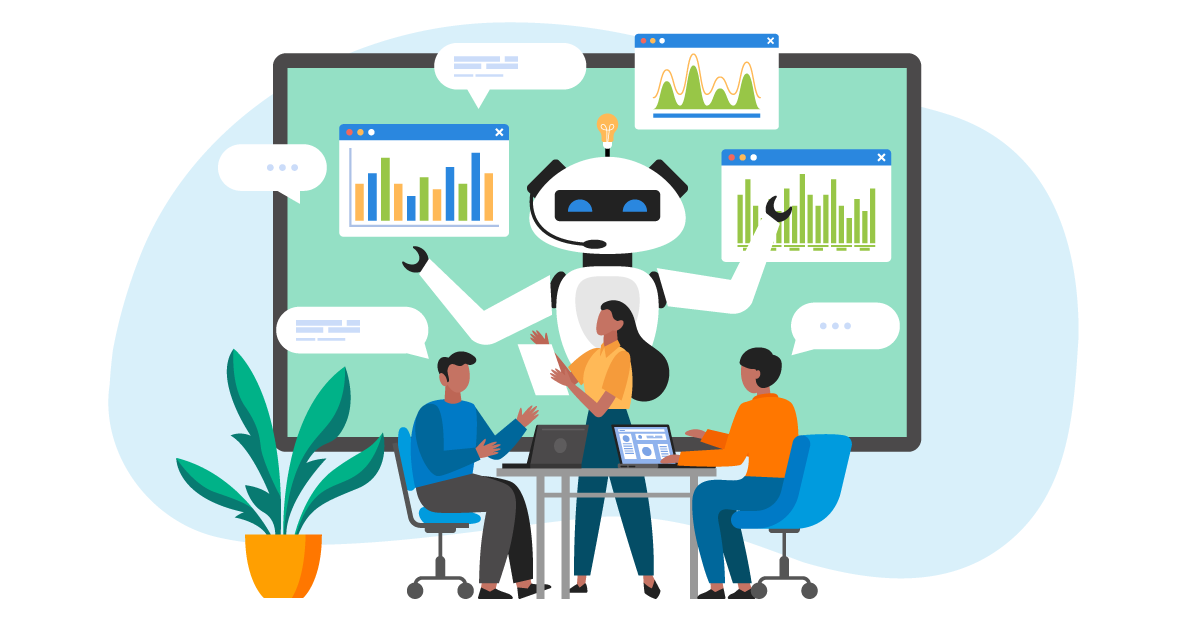
AI Can’t Fix Bad Data. These Ideas Can Get You on the Right Track. Real estate visionaries constantly integrate innovative technology to make their organizations more efficient.
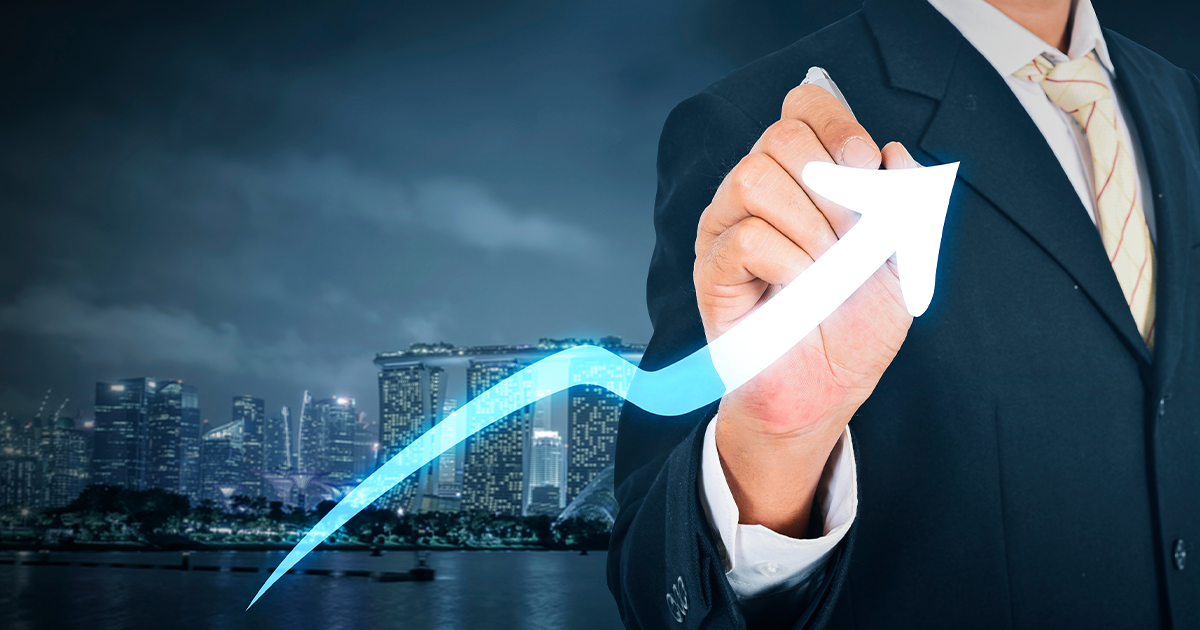
Managing Building Automation and Integration Like an Investment Portfolio What if your building automation and integration decisions were managed with the same precision, discipline, and long-term vision as Warren Buffett’s investment portfolio?
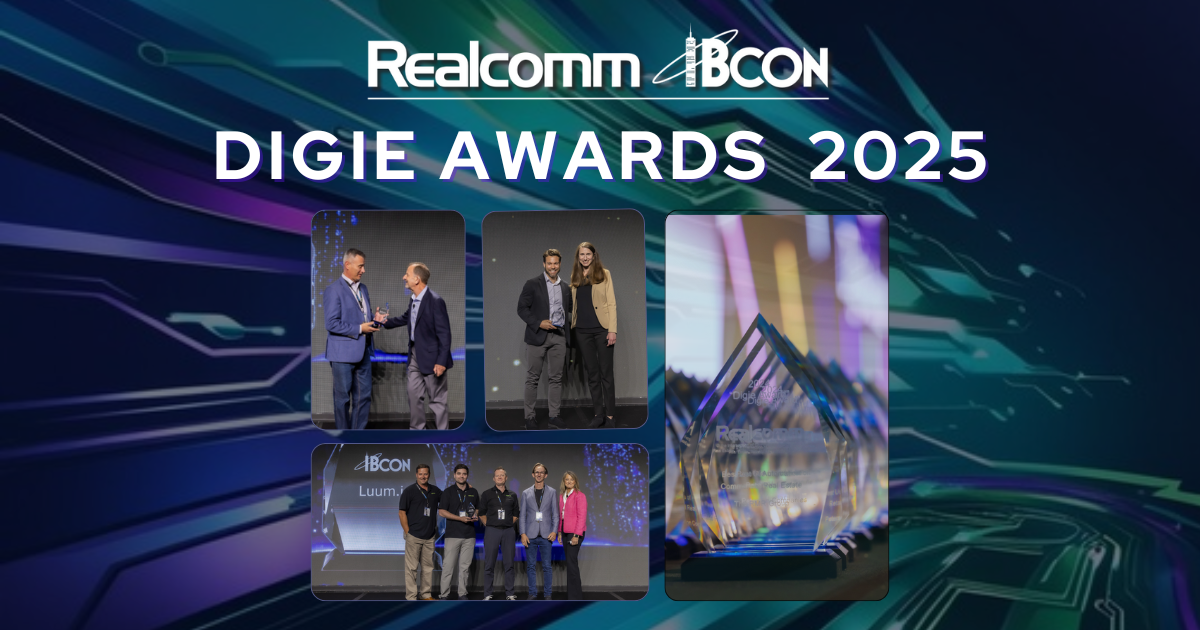
Tech, Talent and Transformation: 2025 Digie Finalists Announced For 27 years, Realcomm has presented the Digie Awards to acknowledge companies, real estate projects, technologies, and individuals that have advanced the commercial real estate industry through the strategic use of technology, automation, and innovation.
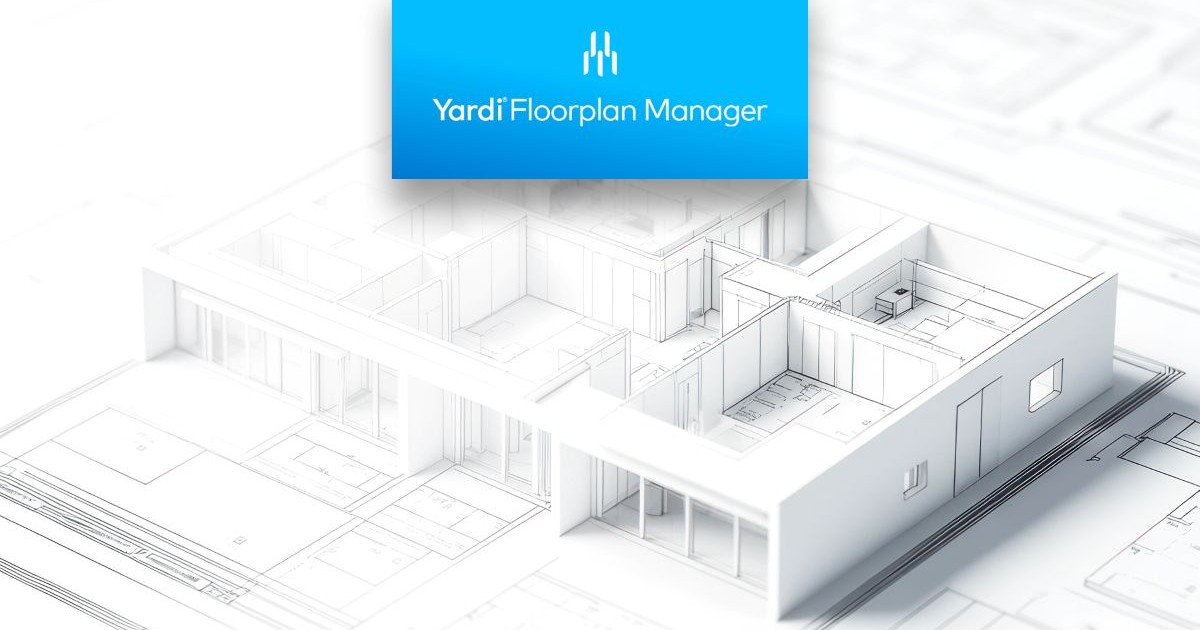
Empowering Space Management with Data-Driven Visualization For effective CRE space management, it’s critical to centralize lease data, maximize rental square footage (RSF), improve energy efficiency and reconfigure spaces to meet changing needs.