How to Think About AI in Real Estate
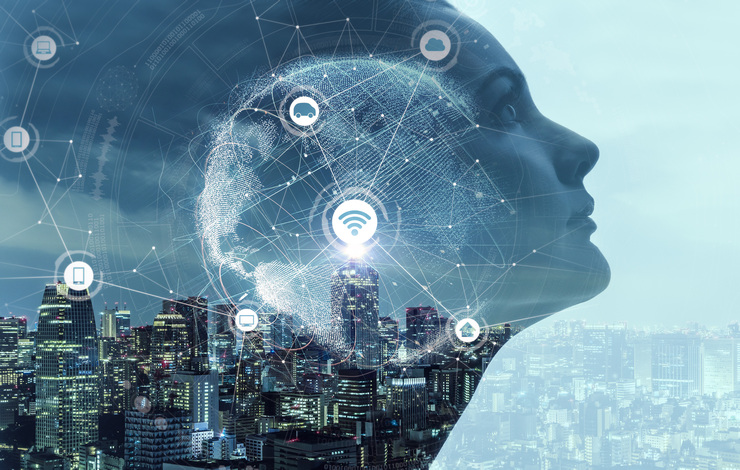
AI, whether we realize it or not, is impacting all our lives in significant ways today. From monitoring our credit card usage for fraud, to filtering our email for spam, to recommending what to watch on Netflix, to recognizing our friends in photographs on Facebook, to flying our planes 95% of the time, to enabling self-driving cars. For many day-to-day processes it is becoming pervasive - behind the scenes.
AI will become pervasive within real estate as well. The extraordinary new capabilities of computers to understand the world around them, to see, hear and read as well as humans, are sure to provide the foundational tools that allow us to build a better built environment.
But how do you need to think about AI in Real Estate? How do you approach a topic which can be (because it is) somewhat overwhelming?
You need to think about AI in Real Estate via a process:
- First you need to understand what AI is good for, and just as importantly what it is not.
- Secondly you need to analyze your workflows and business processes to see where you can leverage what AI is good at.
- Thirdly, you need to consider which of these use cases is commonplace and repeatable within your own business and/or applicable to many of your customers.
- Fourthly, you need to look for use cases where you have or can obtain large quantities of data, and where there are clear metrics by which you can judge success.
- Fifthly and lastly, if you have all of the above, is that something that will create significant value?
Over the last five years AI has developed very rapidly in three key areas: Computer Vision, Voice Recognition and Natural Language Processing. Taking Computer Vision as an example, in 2013 the ImageNet Competition Winner (ImageNet runs annually and quantifies the capabilities of different systems) had an error rate of 11.3%. In 2017 that had come down to 2.2%. For reference, humans rate at 5%. Similar improvements apply to NLP and Voice recognition. In practical terms these three skills have gone from 'Useless to Utility' and this is why we have seen the rapid rise of driverless cars (or semi-autonomous ones like Teslas) and the explosive rise of Amazon's Alexa.
A combination of improved algorithms, vast new data sets to train on, and massive computational power has meant that much that was foretold back in the early days of AI (the term was first coined at a conference at Dartmouth College in 1956) is now possible. And critically, because of the way AI works (essentially recursively - the more you know, the more you can know; and the faster you go, the faster you'll be able to go), over the last five years in particular the pace of development has actually gotten faster.
Which means that, today, AI is very good at:
- Understanding what is happening in pictures and videos
- Understanding people using language
- Creating content (auto generated commentary, extracting data from reports, news etc.)
- Automating processes
- Optimizing complex systems
- Making predictions
In terms of what AI cannot do, the key point to understand is that computers operate within very specific boundaries. Google's Alpha Go (that beat grandmaster Lee Sedol at the famously complex and complicated Chinese board game Go) would be completely useless if you used it to play chess.
It is true that Machine and Deep Learning - both of which are subsets of AI, and are differentiated by being systems that are not explicitly programmed to do A then B then C or D if X = Y - can to an extent 'think for themselves' but they can only do this within very narrow boundaries. Computers are great at optimizing complex systems because that plays entirely to their strengths; but ask a computer to create something completely new that turns out to be complex and it will fail.
Even in an 'AI first' world, as Google boss Sundar Pichai said we were entering last year, a human 'augmented' by a computer will trump any computer on its own. By the same token, a human/computer pairing will also trump a human on their own. To make the most of AI you need to think in terms of what computers are good at and what human skills can be used to leverage those capabilities. We need each other, to be the best we can be, at what we are good at.
Once your thinking is clear about what AI is good at you need to look for use cases. What tasks do you or your company perform that are "Structured, Repeatable or Predictable?" Last year the RICS released a report saying that 88% of the tasks surveyors perform are vulnerable to being automated within 10 years. In 2016, McKinsey said that 49% of ALL tasks people are paid to perform globally are capable of being automated by "currently demonstrable technology." The answer is probably "quite a few."
So, list them out; what do you do that follows a pattern in some way? Either because they absolutely do involve linear processes, or because "with these inputs you know you can produce those outputs." You'll find workflows or processes from across the entire lifecycle of real estate, from planning, to design, construction, leasing, occupation and management and on to portfolio analysis and investment sales or acquisition.
What imagery or video content do you deal with, or what movements around space would be useful to track? What customers do you interact with and how much do you know about them? What reports do you have to generate and how are they put together; could these be automated? How do you handle questions from customers; do these follow a pattern? Etc.
Once prepared, you can move to the third stage. Take your list and give each item two scores of 1 to 10. First, how common is this task in your business; and secondly, how common is it outside of your business, either amongst customers or competitors? You are looking for tasks that are repeated over and over again. What are the primary 'Jobs to be Done' in your area of real estate? This is a proxy for market size in terms of the potential AI service that addresses each task. We're looking to use AI to help us do tasks much faster than now, or much cheaper, or more accurately - so it is sensible to look for those tasks we, or our customers, have to do a lot of.
Now we need to look at data. How much of it about each task do we have? Here you should think of the four Vs: Volume, Variety, Velocity and Veracity. How much data do we have, from how many different sources, how fast is it being generated (as per seconds, hours, days, months, years) and how accurate is it? Regarding accuracy you must be honest; we all know a lot of real estate data is, being polite, somewhat lacking in rigor. If utilized by AI, inaccurate training data will lead to inaccurate results, and it is frankly better not to waste your time with it.
It is not an absolute that more data makes for better AI but in many cases, a lot of solid, accurate data is a great benefit. Also, knowing what you have and what you lack is very useful. You might be able to acquire what you need from third parties but without a good data audit you won't know where to start. Thinking about AI involves a lot of thinking about data.
You need to think about correlation not being causation and apply that to your data. You need to think in terms of "with these data points I could arrive at this conclusion." For example, if I knew exactly how occupiers of my building moved around, I could optimize the AC and lighting. Or, if I knew these characteristics of an investment, I could match them against these characteristics of an investor.
Think big here. Hypothesize about being able to do something that you cannot do today. What data would I need to make that happen? Work on the assumption that if you had said data we now have the tools to analyze it deeply. How could I filter out from thousands or tens of thousands of companies which ones will lease my new office development?
And moving to the fifth stage, "where's the money?" From all that we have looked at which areas, with what solutions, could generate the most value? This might mean saving money, or time, or it might mean creating a much larger market or more valuable products or services. Value comes in many forms.
It will sometimes be the case that "digitizing the past," where you simply apply new technology to existing business processes, makes sense and is worthwhile, but the real value in this field, applying AI, will be in creating products or services that did not exist before. There is little competitive advantage in doing what everyone else can do, so your thinking needs to be focused on the mountaintop beyond the horizon.
By this stage you should be a long way towards that goal.
In conclusion then, thinking about AI in Real Estate involves understanding the new technologies; looking for use cases and determining what use cases are core and ongoing or transitory; getting to grips with data, what you need and whether you have it or can get it; and then homing in on where real value lies.
There is not a shortcut through this process, though it'll probably turn out to be not as daunting as you might imagine. Once you have a structured way to think about this new world, things do tend to fall into place more quickly than you imagine. Utilizing AI is as much a mindset as a technology.
At all times though, what must remain uppermost in your thinking is that whoever really cracks using AI in Real Estate will be orders of magnitude more productive, and profitable, than the non-AI embracing mainstream. AI is potentially truly disruptive.
AI and other emerging technologies will be covered in-depth at Realcomm | IBcon 2019. The event will be held at the Nashville Music City Center on June 13 & 14 (Golf and RE Tech Tours June 11 | Pre-Con Events: June 12). Register today!
This Week’s Sponsor
Corning is one of the world's leading innovators in materials science, with a more than 165-year track record of life-changing inventions. Corning applies its unparalleled expertise in glass science, ceramic science, and optical physics along with its deep manufacturing and engineering capabilities to develop category-defining products that transform industries and enhance people's lives.
Read Next
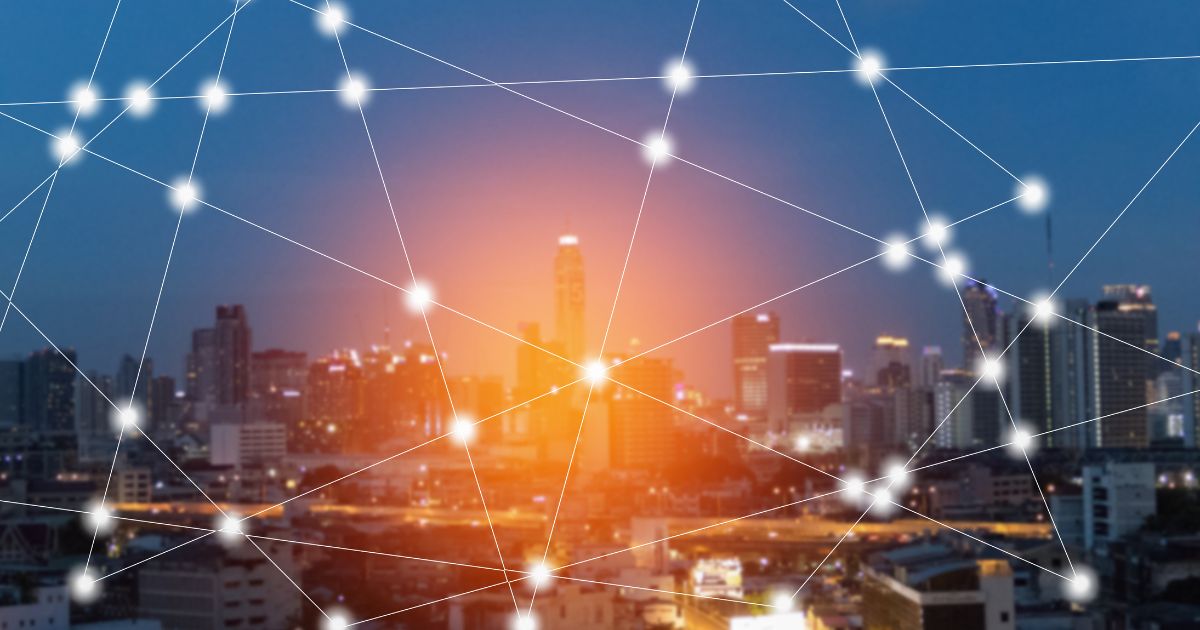
The Convergence of Edge Computing, Cloud, and AI in Building Automation and Smart Buildings In the built environment, we have seen the convergence of Operational Technology (OT) and Information Technology (IT), later expanding to include Workplace Technologies (WP).
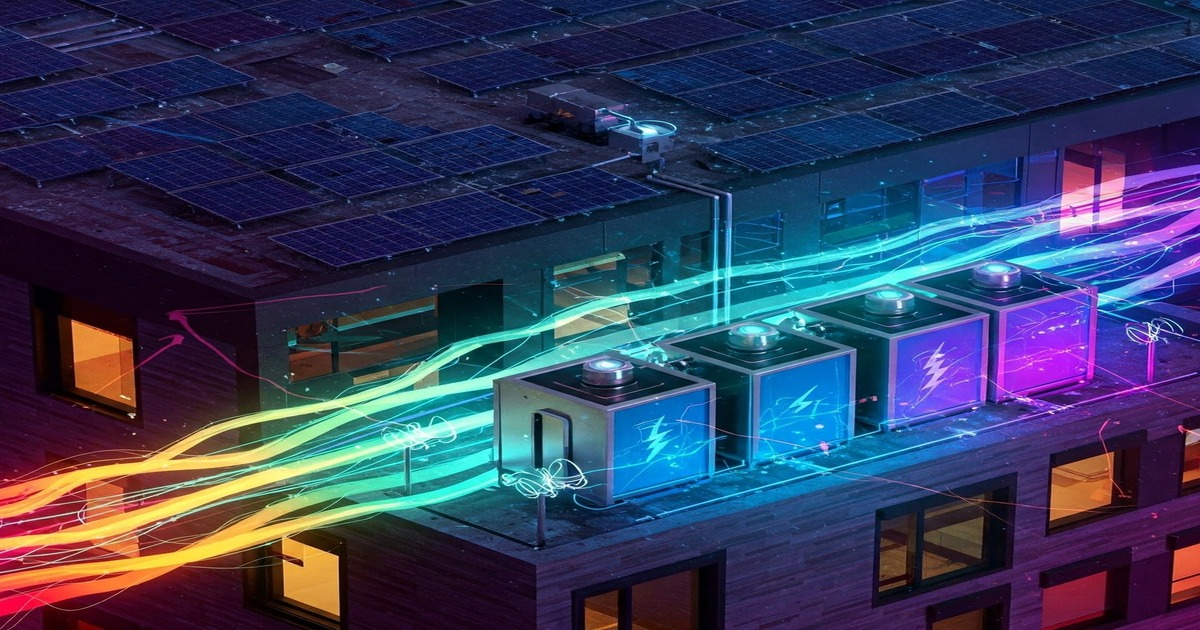
DC Power: A Holistic Approach to Energy Savings in Commercial Buildings In today's energy-conscious world, businesses constantly seek ways to reduce their carbon footprint and operational costs.
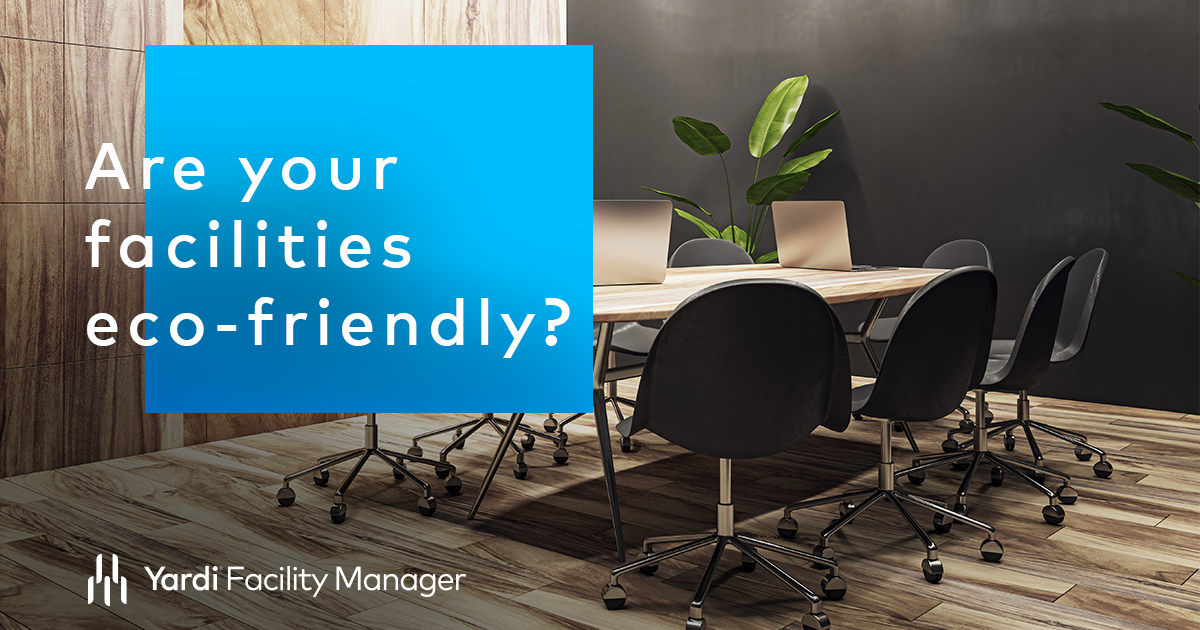
How to Achieve Eco-Friendly Facility Management Commercial real estate operators and facility managers are focusing on sustainable practices to minimize environmental impact, create healthier workplaces, improve productivity and lower operational costs.
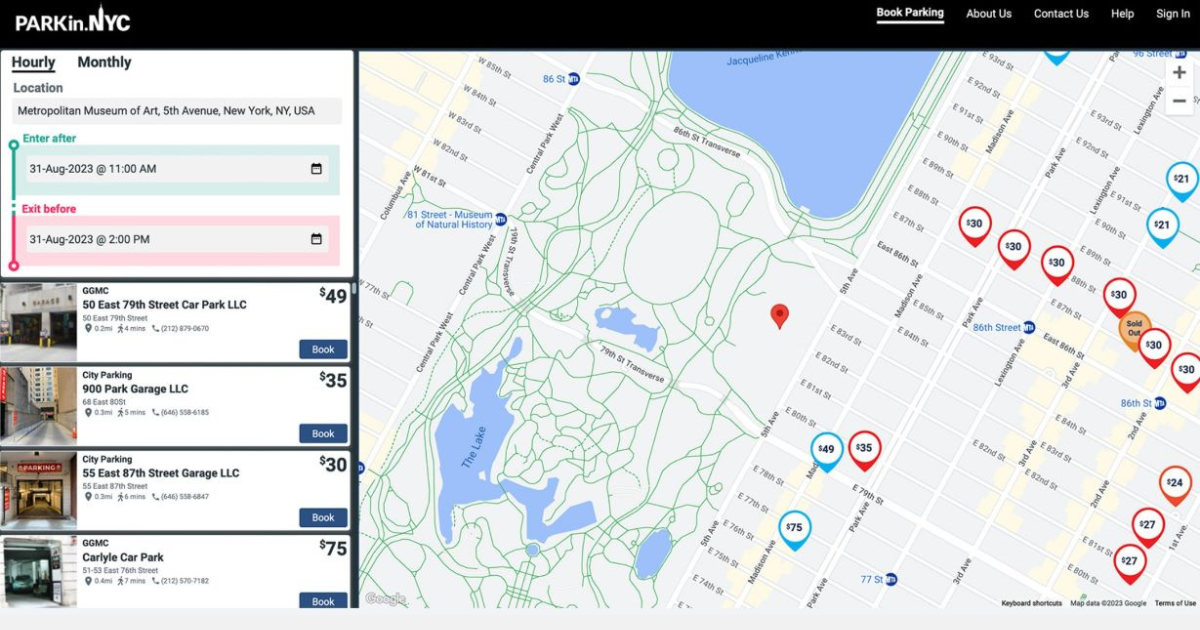
When It Comes To Managing Properties’ Parking, Technology Is Key It’s easy for developers and real estate owners to think of parking as a necessary evil. They know they have to provide it (often, because it’s mandated by code), they understand that prospective tenants and buyers expect to be provided parking, but they haven’t figured out how to maximize its value.