How Mapped Supports Multiple Ontologies for Smart Buildings
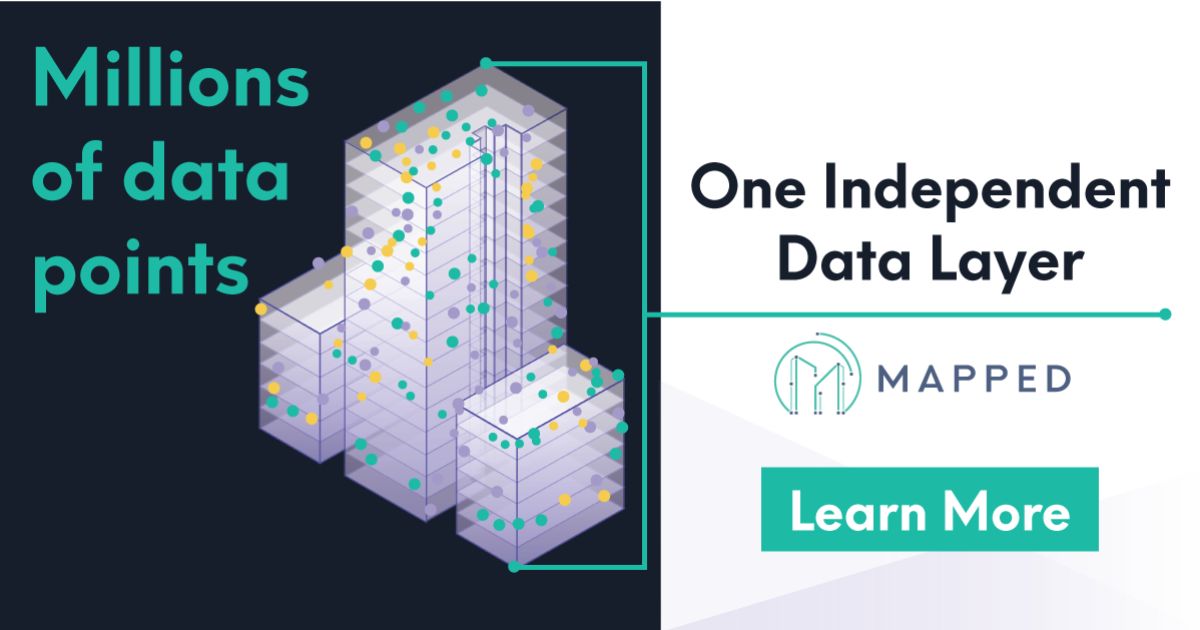
Commercial and industrial buildings across the world are facing significant challenges – managing greenhouse gas emissions, the impact of climate change, smart and hybrid offices becoming more prevalent. Property owners, facility operators, and tenants require data from building systems like HVAC to manage these challenges. Systems inside a building are frequently provided by different vendors, using different protocols, different naming conventions, and different methods to retrieve the data. This makes it very difficult to analyze, integrate, and implement business solutions across buildings and locations.
Implementing an ontology significantly reduces or eliminates the integration challenge. In simplest terms, an ontology defines common vocabularies for concepts, such as applying the label “Temperature Sensor” to data that’s listed as “Temperature,” “Tmp_Sensor” or “Tmp” in actual systems. The common vocabulary applied by an ontology could include type labels, relationship information, and properties which when put together form a graph.
There are several ontologies for modeling building systems – RealEstateCore, Google Digital Buildings, Project Haystack, Matter, Azure Digital Twin, and the BRICK schema (which we use at Mapped). BRICK is an open-source ontology backed by many academic leaders and industry leaders like Johnson Controls, Schneider and Siemens.
We understand it is a significant investment for our customers to choose an ontology for a portfolio, but a well-chosen framework with an ontology opens the door to more possibilities – including integration with other ontologies through “ontology mapping.” Since an ontology is essentially a language with specific rules, each ontology can be translated into another. This ontology mapping process can be repeated for any other ontologies as needed. BRICK in particular was intentionally designed to abstract many variations in domain-specific concepts (e.g., fault detection rules, control sequences, etc.) to act as a lingua franca. The translation may need an expert’s input to map the vocabularies to different ontologies, but it is a one-time effort that can be reused in different instances.
For example, Microsoft’s Azure has an IoT platform called Azure Digital Twins (ADT) and existing Azure users may want to integrate data from Mapped into ADT. ADT uses an ontology called Digital Twins Definition Language (DTDL) and our ontology can be easily mapped to ADT by bridging the same concepts between them – such as DTDL’s “Interface” set to Mapped’s “Entity,” which is also equivalent to BRICK’s “Class. ”To view the full specs of Microsoft’s DTDL ontology mapping, it’s open-sourced and available here.
We’re excited to say we implemented the above DTDL ontology mapping, and Mapped is now available on Microsoft’s AppSource Marketplace. Mapped will be powering Microsoft’s soon-to-be-released Facility Digitization Accelerator, which will enable joint customers to automate and simplify the process of data onboarding and integration, helping accelerate sustainability initiatives.
For more details about Mapped, check out our website and blog.
This Week’s Sponsor
Mapped started with the idea “what if every built space had an API?” Getting data out of buildings was a complex, time consuming and often manual process - weeks or even months of work. To solve the problem, we created a knowledge graph of people, places and things using machine learning to automate the process of data extraction and identification. We then built a simple, secure and unified API on top; now anything that generates data - devices, sensors, enterprise applications and more - is accessible quickly, easily and securely.
Read Next
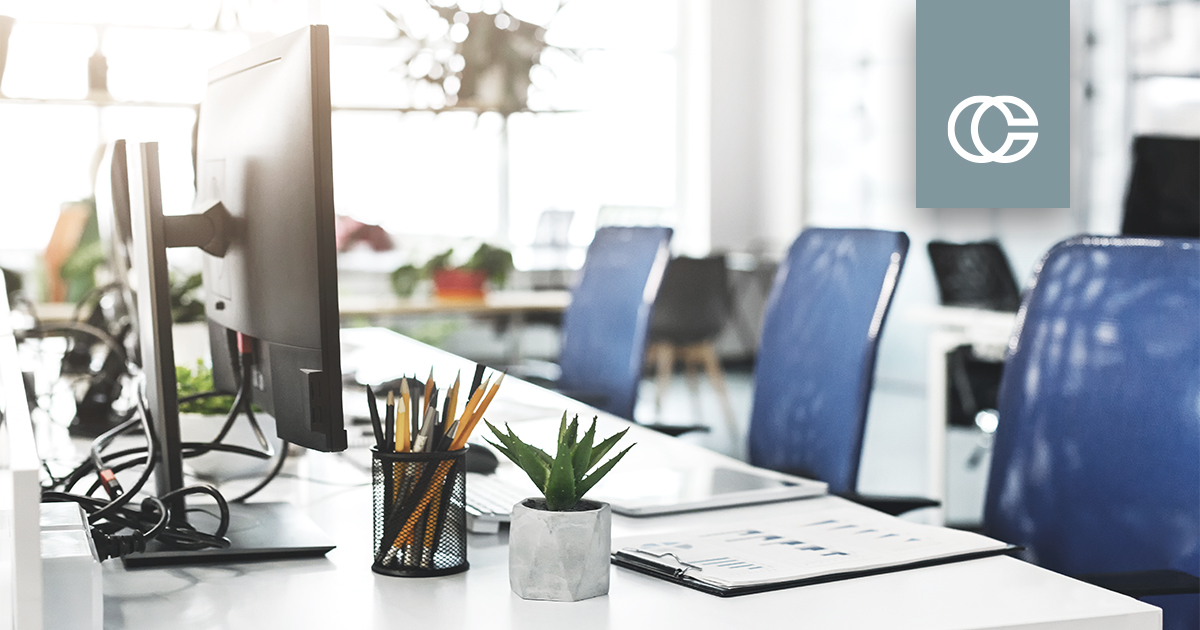
Best Practices for Managing Lease Renewals When your commercial leases come up for renewal, it’s a great opportunity to assess your real estate portfolio, consider the value of current leases and possibly negotiate better terms.
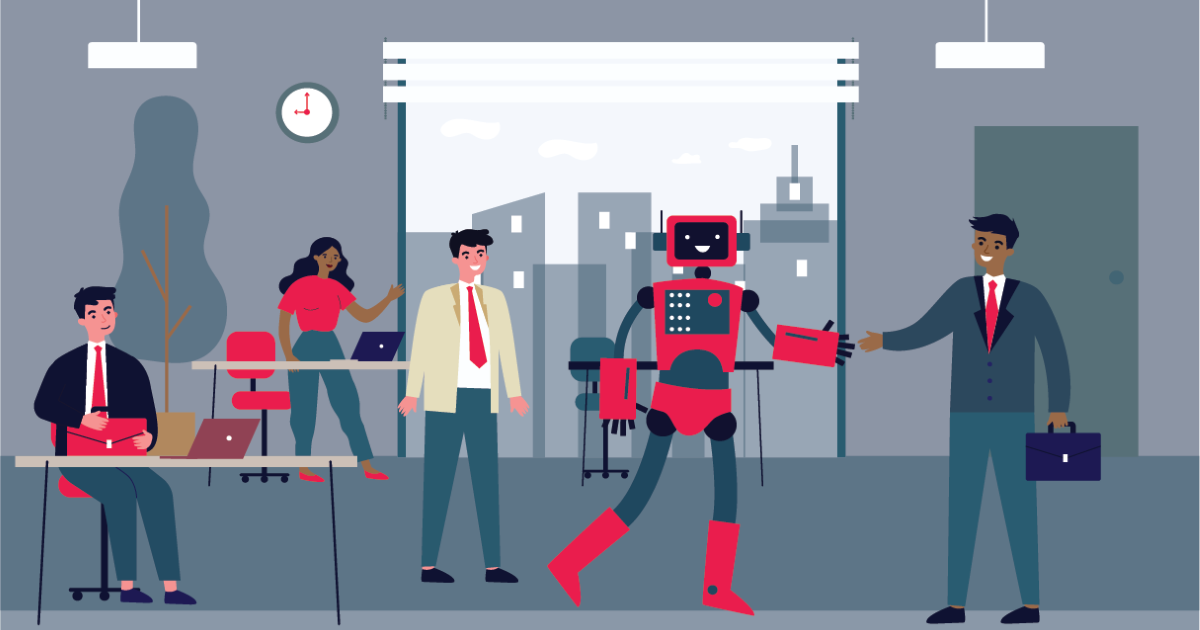
3 Reasons to Incorporate AI Into Your Talent Retention Strategy Introducing new technology into the workplace is often met with suspicion.
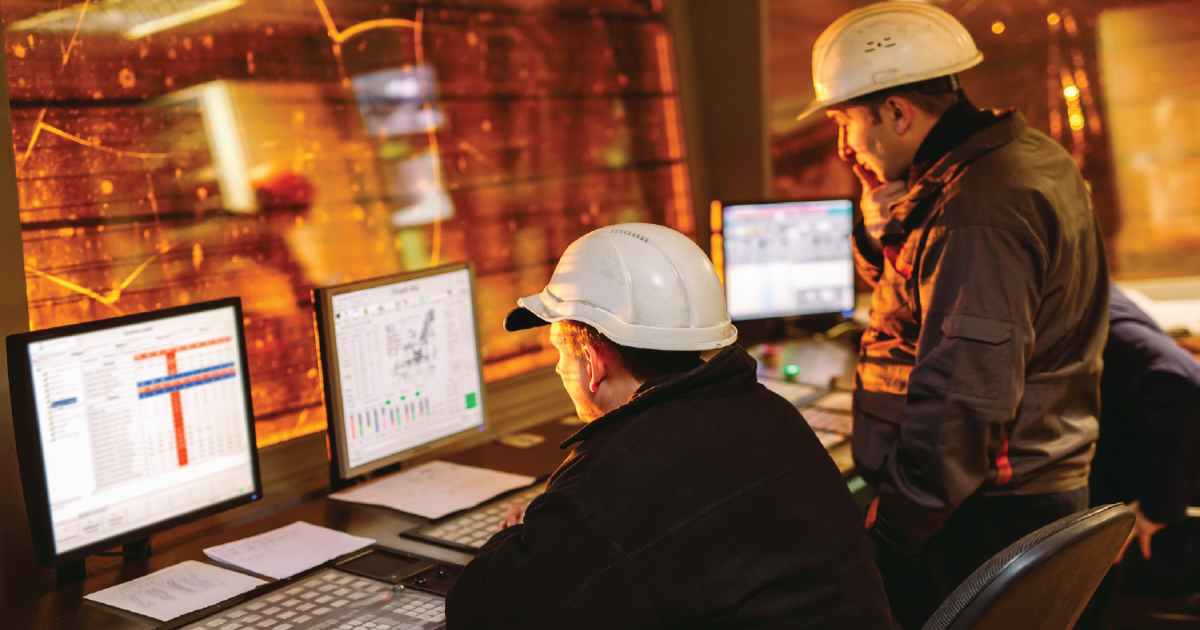
Operational Technology (OT) Data Data has been a cornerstone of business since the early days of computing in the 1960s.
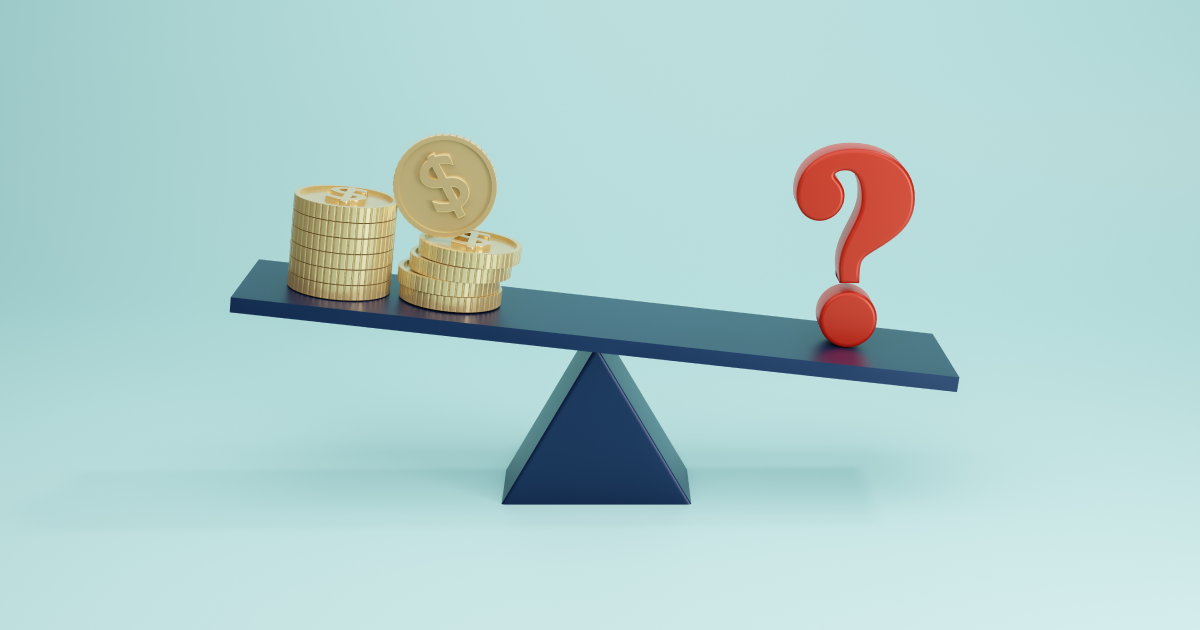
The Tech-Forward Response to Rising CRE Cap Rates is Also People-First Cap rates on commercial real estate have been rising for five consecutive quarters, leading to an estimated 20% drop in value for many property types, according to CBRE’s latest U.S. Real Estate Market Outlook.